Author: Evgeny Bodyagin, https://dsprog.pro
Introduction
So, there is an online store (website). He is already running ad campaign A. There is also an alternative option: to run ad campaign B. Let’s do an A-B test to find out if the effectiveness of these campaigns is different?
Experiment Design
- Null hypothesis: No performance difference between campaigns A and B.
- Alternative hypothesis: There is a significant performance difference between campaigns A and B.
- Processing object: Two samples of site visitor cookies mixed randomly. The sample sizes are the same.
- Target Audience: Website visitors in the last 30 days.
- Metrics: CTR, CR, CPC, CPA
- CTR description: (number of clicks) / (number of impressions) * 100
- CR description: (number of purchases) / (number of clicks) * 100
- CPC description: (spend in USD) / (number of clicks) * 100
- CPA description: (spend in USD) / (number of purchases) * 100
- Duration of the test: 1 month
- Significance levels:
- α = 0.05
- β = 0.2
- Campaigns are launched at the same time, and the audience is divided randomly.
Leveling: Test Significance and Test Power
import scipy # Significance level (probability of Type I error) that is used to decide whether to reject the null hypothesis. alpha = 0.05 # Test power (probability of type II error), which indicates the probability of not detecting a real effect if it exists. beta = 0.2 alpha_zscore = -1 * round(scipy.stats.norm.ppf(1 - alpha/2), 4) beta_zscore = -1 * round(scipy.stats.norm.ppf(1 - beta), 4) print('alpha_zscore: ', alpha_zscore) print('beta_zscore: ', beta_zscore)
alpha_zscore: -1.96 beta_zscore: -0.8416
Determining the Minimum Sample Size
import pandas as pd import numpy as np import matplotlib.pyplot as plt import seaborn as sns from scipy import stats sns.set() # Initial conversion, which is taken as a starting point for comparison with other options: baseline_cr = 0.095 # Minimum detectable effect: min_detectable_effect = 0.005 # this content is from dsprog.pro p2 = baseline_cr + min_detectable_effect n = (((alpha_zscore * np.sqrt(2 * baseline_cr * (1 - baseline_cr))) + (beta_zscore * np.sqrt(baseline_cr * (1 - baseline_cr) + p2 * (1 - p2))))**2) / (abs(p2 - baseline_cr)**2) print('Sample Size: ', round(n, 0))
Sample Size: 54363.0
Data structure
- Advertising campaign (Campaign Name): The name of the advertising campaign.
- Date: Date of the advertising campaign.
- Impressions (of Impressions): Number of ad impressions.
- Reach: Audience coverage, the number of unique users who saw the ad. Allows you to determine how many people learned about advertising.
- Clicks (of Website Clicks): The number of clicks on the banner and went to the site.
- Of View Content: The number of times users viewed content on the site.
- of Add to Cart: The number of times users have added items to their shopping cart.
- Purchases (of Purchase): The number of times users have made a purchase.
- Costs (Spend /USD/): Advertising costs.
- of Searches: The number of times users searched the site after clicking.
Loading data
The experiment was carried out for 1 month. Let’s load the existing data.
a_group = pd.read_csv('input/Xa_group.csv', delimiter=',') a_group['Date'] = pd.to_datetime(a_group['Date'], format='%Y-%m-%d', dayfirst=True) a_group.head()
Campaign Name | Date | # of Impressions | # Reach | # of Website Clicks | # of View Content | # of Add to Cart | # of Purchase | # Spend [USD] | # of Searches | |
---|---|---|---|---|---|---|---|---|---|---|
0 | a campaign | 2023-07-27 | 36908 | 104034 | 1024 | 2485 | 1028 | 521 | 2158 | 2609 |
1 | a campaign | 2023-07-19 | 129930 | 78269 | 2557 | 1568 | 1250 | 882 | 1979 | 1223 |
2 | a campaign | 2023-07-18 | 94853 | 110617 | 3126 | 2719 | 2173 | 922 | 2547 | 1415 |
3 | a campaign | 2023-07-30 | 152514 | 89152 | 3127 | 1955 | 1137 | 218 | 2454 | 2340 |
4 | a campaign | 2023-07-25 | 100917 | 91991 | 3448 | 2056 | 793 | 490 | 2356 | 1474 |
b_group = pd.read_csv('input/Xb_group.csv', delimiter=',') b_group['Date'] = pd.to_datetime(b_group['Date'], format='%Y-%m-%d', dayfirst=True) b_group.head()
Campaign Name | Date | # of Impressions | # Reach | # of Website Clicks | # of View Content | # of Add to Cart | # of Purchase | # Spend [USD] | # of Searches | |
---|---|---|---|---|---|---|---|---|---|---|
0 | b campaign | 2023-07-01 | 79051 | 47257 | 7431 | 1636 | 989 | 707 | 2397 | 2424 |
1 | b campaign | 2023-07-02 | 90101 | 63332 | 6070 | 2208 | 763 | 687 | 1996 | 1733 |
2 | b campaign | 2023-07-03 | 113952 | 52829 | 5376 | 1834 | 934 | 466 | 2223 | 2455 |
3 | b campaign | 2023-07-04 | 73913 | 105952 | 9453 | 3724 | 1416 | 218 | 2974 | 1263 |
4 | b campaign | 2023-07-05 | 59248 | 28222 | 8436 | 959 | 388 | 346 | 2734 | 1258 |
Calculation of metrics
a_group['CTR'] = a_group['# of Website Clicks']/a_group['# of Impressions'] a_group['CR'] = a_group['# of Purchase']/a_group['# of Website Clicks'] a_group['CPC'] = a_group['# Spend [USD]']/a_group['# of Website Clicks'] a_group['CPA'] = a_group['# Spend [USD]']/a_group['# of Purchase'] b_group['CTR'] = b_group['# of Website Clicks']/b_group['# of Impressions'] b_group['CR'] = b_group['# of Purchase']/b_group['# of Website Clicks'] b_group['CPC'] = b_group['# Spend [USD]']/b_group['# of Website Clicks'] b_group['CPA'] = b_group['# Spend [USD]']/b_group['# of Purchase'] # this content is from dsprog.pro a_group.head()
Campaign Name | Date | # of Impressions | # Reach | # of Website Clicks | # of View Content | # of Add to Cart | # of Purchase | # Spend [USD] | # of Searches | CTR | CR | CPC | CPA | |
---|---|---|---|---|---|---|---|---|---|---|---|---|---|---|
0 | a campaign | 2023-07-27 | 36908 | 104034 | 1024 | 2485 | 1028 | 521 | 2158 | 2609 | 0.027745 | 0.508789 | 2.107422 | 4.142035 |
1 | a campaign | 2023-07-19 | 129930 | 78269 | 2557 | 1568 | 1250 | 882 | 1979 | 1223 | 0.019680 | 0.344935 | 0.773954 | 2.243764 |
2 | a campaign | 2023-07-18 | 94853 | 110617 | 3126 | 2719 | 2173 | 922 | 2547 | 1415 | 0.032956 | 0.294946 | 0.814779 | 2.762473 |
3 | a campaign | 2023-07-30 | 152514 | 89152 | 3127 | 1955 | 1137 | 218 | 2454 | 2340 | 0.020503 | 0.069715 | 0.784778 | 11.256881 |
4 | a campaign | 2023-07-25 | 100917 | 91991 | 3448 | 2056 | 793 | 490 | 2356 | 1474 | 0.034167 | 0.142111 | 0.683295 | 4.808163 |
b_group.head()
Campaign Name | Date | # of Impressions | # Reach | # of Website Clicks | # of View Content | # of Add to Cart | # of Purchase | # Spend [USD] | # of Searches | CTR | CR | CPC | CPA | |
---|---|---|---|---|---|---|---|---|---|---|---|---|---|---|
0 | b campaign | 2023-07-01 | 79051 | 47257 | 7431 | 1636 | 989 | 707 | 2397 | 2424 | 0.094003 | 0.095142 | 0.322568 | 3.390382 |
1 | b campaign | 2023-07-02 | 90101 | 63332 | 6070 | 2208 | 763 | 687 | 1996 | 1733 | 0.067369 | 0.113180 | 0.328830 | 2.905386 |
2 | b campaign | 2023-07-03 | 113952 | 52829 | 5376 | 1834 | 934 | 466 | 2223 | 2455 | 0.047178 | 0.086682 | 0.413504 | 4.770386 |
3 | b campaign | 2023-07-04 | 73913 | 105952 | 9453 | 3724 | 1416 | 218 | 2974 | 1263 | 0.127894 | 0.023061 | 0.314609 | 13.642202 |
4 | b campaign | 2023-07-05 | 59248 | 28222 | 8436 | 959 | 388 | 346 | 2734 | 1258 | 0.142385 | 0.041015 | 0.324087 | 7.901734 |
Advertising spending
fig, ax = plt.subplots(ncols=2, figsize=(14,6)) ax1 = sns.kdeplot(a_group['# Spend [USD]'], ax=ax[0], color='green', fill=True) ax2 = sns.kdeplot(b_group['# Spend [USD]'], ax=ax[1], color='green', fill=True) ax1.set_title('a campaign') ax2.set_title('b campaign') plt.show()
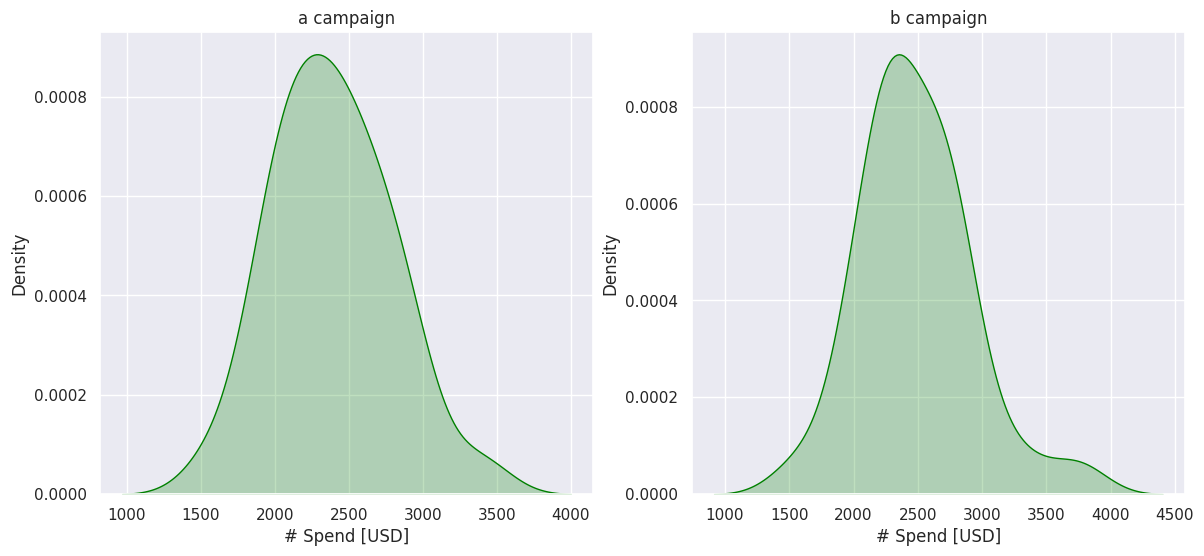
Impressions
fig, ax = plt.subplots(ncols=2, figsize=(14,6)) ax1 = sns.kdeplot(a_group['# of Impressions'], ax=ax[0], color='blue', fill=True) ax2 = sns.kdeplot(b_group['# of Impressions'], ax=ax[1], color='blue', fill=True) ax1.set_title('a group impressions') ax2.set_title('b group impressions') # this content is from dsprog.pro plt.show()
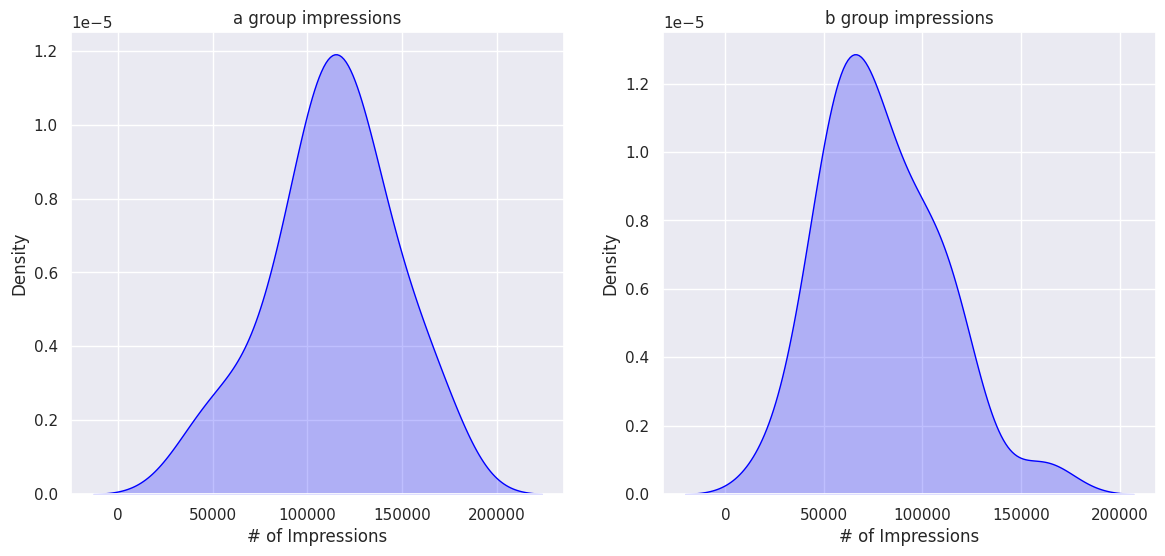
Website Clicks
fig, ax = plt.subplots(ncols=2, figsize=(14,6)) ax1 = sns.kdeplot(a_group['# of Website Clicks'], ax=ax[0], color='gray', fill=True) ax2 = sns.kdeplot(b_group['# of Website Clicks'], ax=ax[1], color='gray', fill=True) ax1.set_title('a group clicks') ax2.set_title('b group clicks') # this content is from dsprog.pro plt.show()
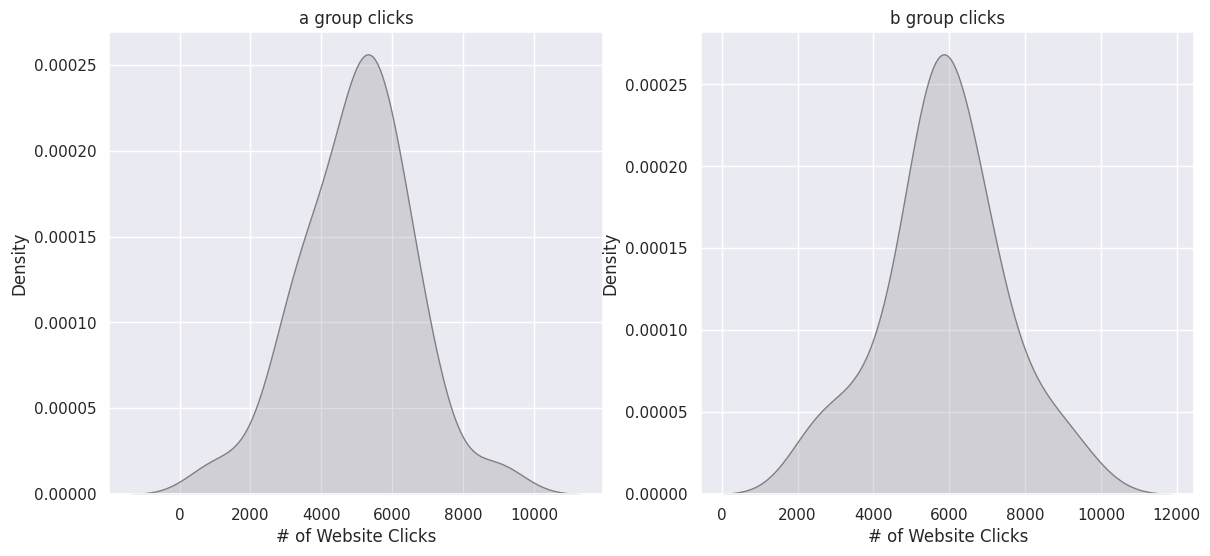
Purchases
fig, ax = plt.subplots(ncols=2, figsize=(14,6)) ax1 = sns.kdeplot(a_group['# of Purchase'], ax=ax[0], color='red', fill=True) ax2 = sns.kdeplot(b_group['# of Purchase'], ax=ax[1], color='red', fill=True) ax1.set_title('a group purchases') ax2.set_title('b group purchases') plt.show()
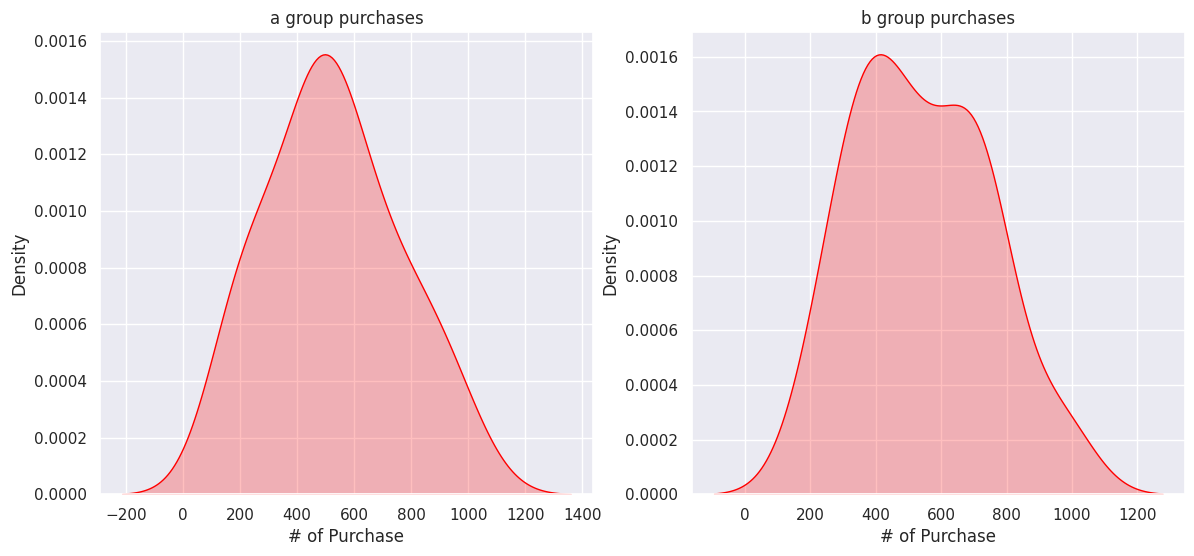
The sample sizes of A and B are comparable and appear to follow a normal distribution.
Descriptive statistics
print(a_group['# of Impressions'].describe()) print(b_group['# of Impressions'].describe())
count 30.000000
mean 113051.066667
std 32856.443638
min 36908.000000
25% 98280.750000
50% 114663.500000
75% 133569.000000
max 173904.000000
Name: # of Impressions, dtype: float64
count 30.000000
mean 79364.766667
std 29599.412623
min 24610.000000
25% 56291.500000
50% 72544.500000
75% 97436.750000
max 162153.000000
Name: # of Impressions, dtype: float64
print(a_group['# of Website Clicks'].describe()) print(b_group['# of Website Clicks'].describe())
count 30.000000
mean 4994.633333
std 1561.157185
min 1024.000000
25% 3863.750000
50% 5100.500000
75% 5734.250000
max 8928.000000
Name: # of Website Clicks, dtype: float64
count 30.000000
mean 5865.633333
std 1593.176192
min 2472.000000
25% 5275.500000
50% 5829.500000
75% 6805.250000
max 9453.000000
Name: # of Website Clicks, dtype: float64
print(a_group['# of View Content'].describe()) print(b_group['# of View Content'].describe())
count 30.000000
mean 1809.933333
std 783.556874
min 104.000000
25% 1508.750000
50% 1906.000000
75% 2204.000000
max 3361.000000
Name: # of View Content, dtype: float64
count 30.000000
mean 1873.033333
std 801.055144
min 100.000000
25% 1615.000000
50% 1816.000000
75% 2430.000000
max 3724.000000
Name: # of View Content, dtype: float64
Analysis of results
desired_palette = ["#0099cc", "#009900"] data_all = pd.concat([a_group, b_group]) fig, ax = plt.subplots(ncols=5, figsize=(34,6)) ax1 = sns.barplot(data=data_all, x='Campaign Name', y='# of Impressions', errorbar=('ci', False), ax=ax[0], estimator='sum', palette=desired_palette) ax2 = sns.barplot(data=data_all, x='Campaign Name', y='# of Website Clicks', errorbar=('ci', False), ax=ax[1], estimator='sum', palette=desired_palette) ax3 = sns.barplot(data=data_all, x='Campaign Name', y='# of View Content', errorbar=('ci', False), ax=ax[2], estimator='sum', palette=desired_palette) ax4 = sns.barplot(data=data_all, x='Campaign Name', y='# of Purchase', errorbar=('ci', False), ax=ax[3], estimator='sum', palette=desired_palette) ax5 = sns.barplot(data=data_all, x='Campaign Name', y='# Spend [USD]', errorbar=('ci', False), ax=ax[4], estimator='sum', palette=desired_palette) ax1.set_title('Impressions') ax2.set_title('Clicks') ax3.set_title('of View Content') ax4.set_title('Purchase') ax5.set_title('Spend [USD]') plt.show()
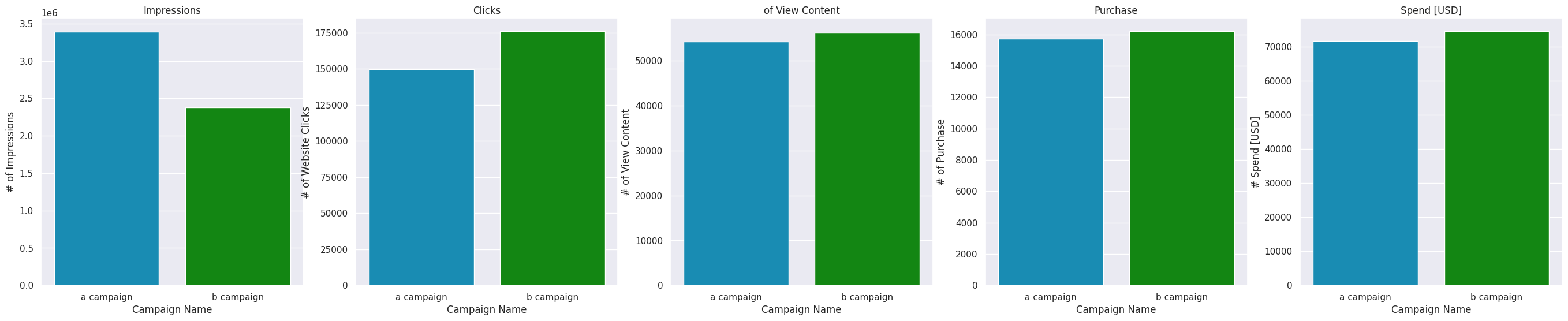
Clearly, Campaign B is more effective than Campaign A. With fewer impressions, Campaign B has more clicks and slightly more sales. It brings more user engagement in viewing the content of the site. At the same time, campaign B is slightly more expensive than campaign A.
Consider also derived indicators.
desired_palette = ["#0099cc", "#009900"] data_all = pd.concat([a_group, b_group]) fig, ax = plt.subplots(ncols=4, figsize=(22,6)) ax1 = sns.barplot(data=data_all, x='Campaign Name', y='CTR', errorbar=('ci', False), ax=ax[0], estimator='mean', palette=desired_palette) ax2 = sns.barplot(data=data_all, x='Campaign Name', y='CR', errorbar=('ci', False), ax=ax[1], estimator='mean', palette=desired_palette) ax3 = sns.barplot(data=data_all, x='Campaign Name', y='CPC', errorbar=('ci', False), ax=ax[2], estimator='mean', palette=desired_palette) ax4 = sns.barplot(data=data_all, x='Campaign Name', y='CPA', errorbar=('ci', False), ax=ax[3], estimator='mean', palette=desired_palette) # this content is from dsprog.pro ax1.set_title('CTR') ax2.set_title('CR') ax3.set_title('CPC') ax4.set_title('CPA') plt.show()
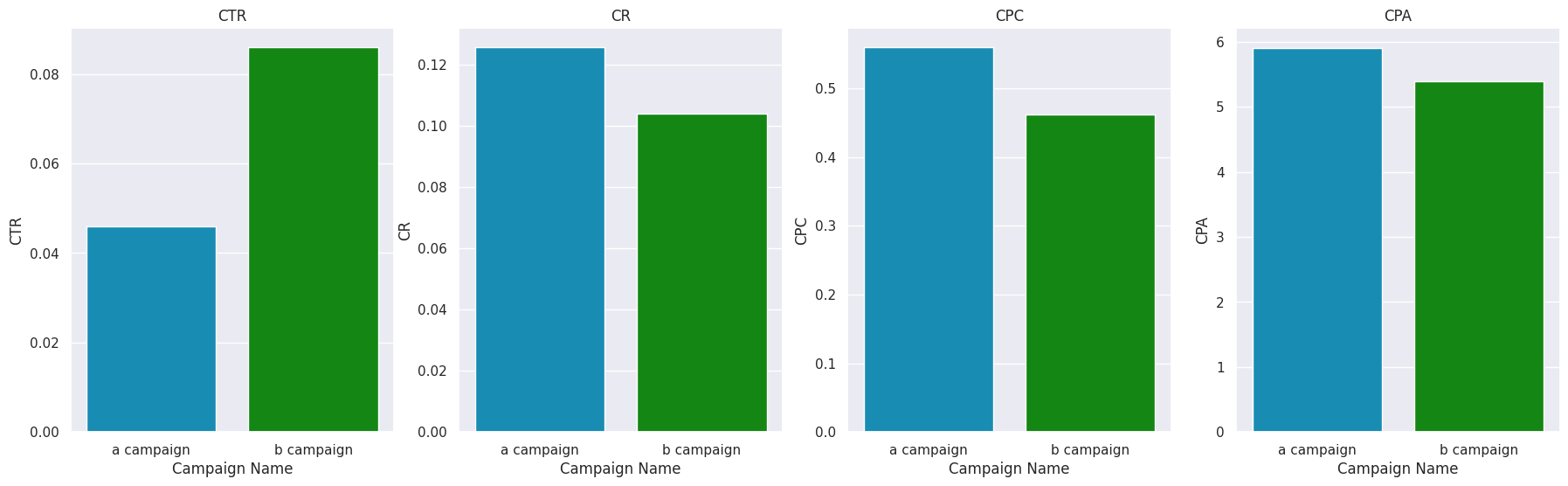
Campaign B had a much higher CTR ((clicks) / (impressions) * 100). Campaign B has a CR ((number of purchases) / (number of clicks) * 100) lower than that of campaign A. This looks a little strange. Campaign A might seem more attractive. But… notice that Campaign B has much more clicks, and Campaign B has a slightly bigger difference in sales. This explains this oddity. The CRC indicator ((costs in rubles) / (number of clicks) * 100) has the same feature as CR. The CPA ((Cost in RUB) / (Number of Purchases) * 100) indicates that Campaign B is more cost-effective.
We use statistical tests to account for differences in data; combined with significance levels (for greater confidence in the results). To do this, we will form the functions of confidence intervals:
- def ratio_diff_confidence_interval(metric, measure_a, measure_b, alpha)
- def cont_diff_confidence_interval(metric, alpha)
Let’s check if the difference in various metrics between the two campaigns is statistically significant.
CTR
ratio_diff_confidence_interval(metric='CTR', measure_a='# of Website Clicks', measure_b='# of Impressions', alpha=.05)
The difference in CTR between groups is 0.0297 Confidence Interval: [ 0.0293 , 0.0301 ]
Reject Null Hypothesis, statistically significant difference between a_group and b_group CTR
CR
ratio_diff_confidence_interval(metric='CR', measure_a='# of Purchase', measure_b='# of Website Clicks', alpha=.05)
The difference in CR between groups is -0.0129 Confidence Interval: [ -0.015 , -0.0109 ]
Reject Null Hypothesis, statistically significant difference between a_group and b_group CR
CPC
cont_diff_confidence_interval(metric='CPC', alpha=.05)
The difference in CPC between groups is -0.0981 Confidence Interval: [ -0.2377 , 0.0414 ]
Unable to reject Null Hypothesis, no statistically significant difference between a_group and b_group CPC
CPA
cont_diff_confidence_interval(metric='CPA', alpha=.05)
The difference in CPA between groups is -0.5102 Confidence Interval: [ -2.1616 , 1.1413 ]
Unable to reject Null Hypothesis, no statistically significant difference between a_group and b_group CPA
Conclusions
With fewer impressions, Campaign B has more clicks and slightly more sales. It brings more user engagement in viewing the content of the site. At the same time, campaign B is slightly more expensive than campaign A.
Campaign B had a much higher CTR ((clicks) / (impressions) * 100). Campaign B has a CR ((number of purchases) / (number of clicks) * 100) lower than campaign A. It looks a little strange. Campaign A might seem more attractive. But… notice that Campaign B has much more clicks, and Campaign B has a slightly bigger difference in sales. This explains this oddity. The CRC indicator ((costs in rubles) / (number of clicks) * 100) has the same feature as CR. The CPA ((Spend in USD) / (Number of Purchases) * 100) indicates that Campaign B is more cost-effective.
You can also draw the following conclusions: Based on the CTR, CR it is possible to reject the hypothesis that the effects of campaign A and campaign B are equal. The reason here is the large difference in the number:
- Impressions;
- clicks (of Website Clicks). Based on CPC scores, CPA cannot reject the hypothesis that the effects of Campaign A and Campaign B are equal. The reason for this is the small difference in numbers:
- expenses (Spend |USD|);
- Purchases (of Purchase).
As a result, we can conclude that campaign B is generally more effective than campaign A.
=======================
For the full content of this article; for cooperation with the author, write to Telegram: cryptosensors
The full content of this article includes files/data:
- Jupyter Notebook file (ipynb),
- dataset files (csv or xlsx),
- unpublished code elements, nuances.
Distribution of materials from dsprog.pro is warmly welcome (with a link to the source).